DistOS-2011W Cassandra and Hamachi: Difference between revisions
(40 intermediate revisions by the same user not shown) | |||
Line 1: | Line 1: | ||
=In the beginning= | =In the beginning= | ||
The Internet has seen remarkable growth over the last few years, both technologically and socially. The demand for real-time information has increased at exponential rates and has put existing information systems to the test. For this paper I decided to look at two new software projects that have emerged over the last few years: Cassandra and Hamachi. Cassandra is a distributed database that evolved from the needs of websites such as Twitter and Facebook, whose need for frequent and short updates was too taxing on standard RDBMSs. Hamachi, which is in part a commercial project now, started as an open-source project aimed at creating zero-config VPNs. The project is now part of LogMeIn but is still free to use for non-commercial purposes. | The Internet has seen remarkable growth over the last few years, both technologically and socially. The demand for real-time information has increased at exponential rates and has put existing information systems to the test. For this paper I decided to look at two new software projects that have emerged over the last few years: [[#Cassandra|Cassandra]] and [[#Hamachi|Hamachi]]. Cassandra is a distributed database that evolved from the needs of websites such as Twitter and Facebook, whose need for frequent and short updates was too taxing on standard RDBMSs. Hamachi, which is in part a commercial project now, started as an open-source project aimed at creating zero-config VPNs. The project is now part of LogMeIn but is still free to use for non-commercial purposes. | ||
In normal implementation circumstances you would not find these two projects paired together, but the concept of having a distributed database over a reasonably secure connection was one I thought was worth exploring. Over the course of this paper I'll discuss both projects in a reasonable amount of detail before detailing my implementation experiment and experience. | In normal implementation circumstances you would not find these two projects paired together, but the concept of having a distributed database over a reasonably secure connection was one I thought was worth exploring. Over the course of this paper I'll discuss both projects in a reasonable amount of detail before detailing my implementation experiment and experience. | ||
=The Idea= | |||
My idea is to explore the feasibility of a near zero-config secure distributed database. Fully realized the idea of having a custom distribution of Linux that users could install on their hardware and be automatically joined onto a database distributed across thousands of machines and thousands of users. | |||
= Cassandra = | = Cassandra = | ||
Line 13: | Line 16: | ||
== The Data Model == | == The Data Model == | ||
[[File:Column_Family.PNG|300px|thumb|left|Cassandra Column Family]] | [[File:Column_Family.PNG|300px|thumb|left|Cassandra Column Family ([[#References|Source #1]])]] | ||
Cassandra takes a different approach compared to RDBMSs when it comes to how data is conceptualized and managed. In a standard RDBMS, data is ''normalized'' into a series of '''tables''' with a set number of columns. For the most part, the types and number of these columns will only rarely change as the need arises. For a later analogy, we'll refer to this model as a "Narrow Row" model. Cassandra operates on a system of '''''Column Families''''' which are analogous to a '''tables''' in an RDBMS, but is much more flexible. Column Families (hereafter referred to as ''CFs'') are a loose grouping of common data keyed by some value for each row in question. However, as opposed to the static feel of a RDBMS table, the number of columns or even the types of columns can and will change under Cassandra. That isn't to say there is no form of schema in Cassandra, because there is a simple type-enforcement schema system in place for each ''CF''. To complete the analogy, the model for Cassandra can be seen as "Wide Row", with the number of columns growing however you please. Different rows in the same CF can even have a different number of columns. | Cassandra takes a different approach compared to RDBMSs when it comes to how data is conceptualized and managed. In a standard RDBMS, data is ''normalized'' into a series of '''tables''' with a set number of columns. For the most part, the types and number of these columns will only rarely change as the need arises. For a later analogy, we'll refer to this model as a "Narrow Row" model. Cassandra operates on a system of '''''Column Families''''' which are analogous to a '''tables''' in an RDBMS, but is much more flexible. Column Families (hereafter referred to as ''CFs'') are a loose grouping of common data keyed by some value for each row in question and are grouped together into '''Keyspaces'''. However, as opposed to the static feel of a RDBMS table, the number of columns or even the types of columns can and will change under Cassandra. That isn't to say there is no form of schema in Cassandra, because there is a simple type-enforcement schema system in place for each ''CF''. To complete the analogy, the model for Cassandra can be seen as "Wide Row", with the number of columns growing however you please. Different rows in the same CF can even have a different number of columns. Internally, Cassandra uses a "always appending" model when writing data to disk, similar to Google's Bigtable or GFS. | ||
The downside to such a data model is that Cassandra has no actual query language. There is no equivalent to SQL in Cassandra. There is also no support for referential integrity, either. With such circumstances maintaining data normalization while keeping processing time to a minimum is extremely difficult and is actively frowned upon. That's right, data duplication is encouraged when working with Cassandra. Luckily, the latest version (0.7.0 as of this writing) has introduced ''indexes'' to the meta-data that can be assigned to a column. This allows for basic boolean logic queries can be constructed to filter results better. | The downside to such a data model is that Cassandra has no actual query language. There is no equivalent to SQL in Cassandra. There is also no support for referential integrity, either. With such circumstances maintaining data normalization while keeping processing time to a minimum is extremely difficult and is actively frowned upon. That's right, data duplication is encouraged when working with Cassandra. Luckily, the latest version (0.7.0 as of this writing) has introduced ''indexes'' to the meta-data that can be assigned to a column. This allows for basic boolean logic queries can be constructed to filter results better. | ||
Line 23: | Line 26: | ||
While Cassandra can operate on a single machine the power lies in setting up a Cassandra cluster. Each instance is referred to as a ''node'' within the cluster. In Cassandra nomenclature the nodes form a ''ring'' and divide the keyspace amongst all of the nodes based on a specified partitioner. There are a number of partitioners available, including some that take physical location into account. | While Cassandra can operate on a single machine the power lies in setting up a Cassandra cluster. Each instance is referred to as a ''node'' within the cluster. In Cassandra nomenclature the nodes form a ''ring'' and divide the keyspace amongst all of the nodes based on a specified partitioner. There are a number of partitioners available, including some that take physical location into account. | ||
= | == Fault Tolerance == | ||
Cassandra employs two protocols that make up it's fault tolerance system, '''Gossip''' and '''Handoffs'''. Gossip is an extension of the usual Heartbeat model of monitoring other servers, and involves a full TCP style SYN/ACK/SYN+ACK process to verify the alive-ness of another node in the ring. When a node attempts to contact another and fails, it "Gossips" this fact to the others. Using a special accrual method for detecting downed nodes the ring can keep track of problem areas and adjust accordingly. The second half of this fault tolerance suite is the '''Handoff''' protocol. When a write destined for a downed node is received by the ring, other nearby nodes will accumulate and keep these entries until the downed node is restored. During startup a node will check if any outstanding handoff messages are destined for it and import them promptly. | |||
= | == Replication == | ||
One of the nice features of Cassandra is a built-in Replication system. When a Keyspace is created the ''replication factor'' dictates how many copies of column data will be spread out over the ring. When writing or reading data to the nodes the clients can specify the desired consistency level that is to be observed relative to the Keyspace's replication factor. Like most distributed databases, Cassandra purports ''eventual'' consistency. | |||
= | === Consistency === | ||
;ONE consistency | |||
: The client will block until the first node available responds to the request. | |||
;QUORUM consistency | |||
: The client will block until a majority of nodes agree on the data to return. | |||
;ALL consistency | |||
: The client will block until all nodes would return the same data | |||
I'll give an example of the impacts of the various replication levels later in my implementation report. | |||
= | == Other options == | ||
Cassandra shares some common ground with a number of other projects: | |||
* Bigtable | |||
* Dynamo | |||
* Riak | |||
* CouchDB | |||
= Hamachi = | |||
=References= | Hamachi is a project aimed at easing the complication of creating and managing a Virtual Private Network. VPNs have historically been used to join external machines onto private networks. A travelling salesman or branch office might use one to gain access to a company's internal resources over possibly insecure lines. With a properly configured VPN encrypted traffic is tunneled over an insecure connection without concern that the contents could be inspected enroute. Hamachi has its origins in the modern playing of classic-era games that don't support WAN based gameplay. By creating a VPN with friends the game would be unable to tell that the machines were not all physically together. | ||
== Caveat == | |||
While the original implementation of Hamachi[[#References|<sup>2</sup>]] was open source the project is currently part of a commercial vendor called [http://logmein.com| LogMeIn]. The reason I chose to use this project was simply to fulfill a need for a quick VPN setup with a minimal amount of fuss. The performance and security of my implementation project would undoubtably be improved by an open source and mediator-free VPN solution such as [http://openvpn.net/| OpenVPN]. Because of the closed source nature of Hamachi I won't be able to comment much about the implementation details, it was simply a means to an end. | |||
== The Basics == | |||
Once the Hamachi client is installed, any client can simply create a network for others to join. Each client is assigned an address in the 5.0.0.0/8 IP range, which up until November 2010 was previously un-routable. This range was selected so as to not interfere with existing local network ranges. The client then, with the assistance of a central server, creates secure tunnels to every other client in the VPN. These tunnels support both TCP and UDP traffic, as well as broadcasting. | |||
= The Project = | |||
My idea was to combine these two separate projects together to create the semblance of a secure distributed database. In normal circumstances Cassandra nodes are expected to be located in a closed private network or to have a difficult-to-maintain VPN set up for them exclusively. | |||
== The Setup == | |||
For the purposes of this project I've created a demonstration project that tests the impact of both the inherent overhead from the VPN as well as the latency of physical distribution. The nodes are defined as follows: | |||
[[File:CH_Network_Layout.png|300px|thumb|borderless|right|4000 demonstration setup]] | |||
=== The Nodes === | |||
; Local Network | |||
: Intersect (running OSX 10.6) | |||
: Cybercontrol (running Ubuntu 10.10) | |||
; Remote Network | |||
: Manos (running Debian Squeeze) | |||
All intra-node communication was over the VPN connection and the benchmarks were run through an API server running on '''Intersect'''. The Internal and External networks were separated physically by around 30km of prime Canadian internet. | |||
=== Configuring Cassandra === | |||
I installed Cassandra 0.7 using [http://wiki.apache.org/cassandra/DebianPackaging| the official Debian repository] for both ''Cybercontrol'' and ''Manos''. Both of these servers were configured to use ''Intersect'' as the '''''seed node''''', the node (or nodes) that would be responsible for bootstrapping new nodes joining the ring. All the configuration that was required to get the nodes talking was to use the Hamachi IP assigned to ''Intersect'' and that was it. | |||
=== The Schema === | |||
I used the following schema for the benchmarking. In it I define 3 keyspaces with 1 column family each. The primary difference between the three keyspaces is the [[#Replication|replication factor]] assigned to each one. The keyspaces are: | |||
; ONE_REP | |||
: Single replication keyspace with a column family named <code>Somedata</code> | |||
; TWO_REP | |||
: Dual replication keyspace with a column family named <code>MoreData</code> | |||
; THREE_REP | |||
: Triple replication keyspace with a column family named <code>EvenMoreData</code> | |||
This schema is in [[#References|YAML]] format and is compatible with Cassandra 0.7 only. | |||
<pre> | |||
keyspaces: | |||
- column_families: | |||
- column_type: Standard | |||
comment: '' | |||
compare_with: org.apache.cassandra.db.marshal.BytesType | |||
default_validation_class: org.apache.cassandra.db.marshal.BytesType | |||
gc_grace_seconds: 864000 | |||
key_cache_save_period_in_seconds: 3600 | |||
keys_cached: 200000.0 | |||
max_compaction_threshold: 32 | |||
memtable_flush_after_mins: 60 | |||
min_compaction_threshold: 4 | |||
name: Somedata | |||
read_repair_chance: 1.0 | |||
row_cache_save_period_in_seconds: 0 | |||
rows_cached: 0.0 | |||
name: ONE_REP | |||
replica_placement_strategy: org.apache.cassandra.locator.SimpleStrategy | |||
replication_factor: 1 | |||
- column_families: | |||
- column_type: Standard | |||
comment: '' | |||
compare_with: org.apache.cassandra.db.marshal.BytesType | |||
default_validation_class: org.apache.cassandra.db.marshal.BytesType | |||
gc_grace_seconds: 864000 | |||
key_cache_save_period_in_seconds: 3600 | |||
keys_cached: 200000.0 | |||
max_compaction_threshold: 32 | |||
memtable_flush_after_mins: 60 | |||
min_compaction_threshold: 4 | |||
name: MoreData | |||
read_repair_chance: 1.0 | |||
row_cache_save_period_in_seconds: 0 | |||
rows_cached: 0.0 | |||
name: TWO_REP | |||
replica_placement_strategy: org.apache.cassandra.locator.SimpleStrategy | |||
replication_factor: 2 | |||
- column_families: | |||
- column_type: Standard | |||
comment: '' | |||
compare_with: org.apache.cassandra.db.marshal.BytesType | |||
default_validation_class: org.apache.cassandra.db.marshal.BytesType | |||
gc_grace_seconds: 864000 | |||
key_cache_save_period_in_seconds: 3600 | |||
keys_cached: 200000.0 | |||
max_compaction_threshold: 32 | |||
memtable_flush_after_mins: 60 | |||
min_compaction_threshold: 4 | |||
name: EvenMoreData | |||
read_repair_chance: 1.0 | |||
row_cache_save_period_in_seconds: 0 | |||
rows_cached: 0.0 | |||
name: THREE_REP | |||
replica_placement_strategy: org.apache.cassandra.locator.SimpleStrategy | |||
replication_factor: 3 | |||
</pre> | |||
== The Benchmark == | |||
For the purposes of this experiment I wrote a simple script to test the responsiveness of a client reading and writing to each of the three keyspaces defined above. Each read or write performed by the script was done with the [[#Consistency|ALL consistency level]], meaning that the call will block until all possible replications have the latest data.The benchmark script ran the following stages with various payload sizes 50 times, averaging the response time for each step: | |||
* Stage 1 | |||
## Remove the test row if it already exists | |||
## Write a single column with the payload bytes to the row | |||
## Read the row back | |||
* Stage 2 | |||
## Remove the test row if it already exists | |||
## Write 50 columns, each having the payload bytes, to the row | |||
## Read the row back | |||
* Stage 3 | |||
## Remove the test row if it already exists | |||
## Write 200 columns, each having the payload bytes, to the row | |||
## Read the row back | |||
* Stage 4 | |||
## Remove the test row if it already exists | |||
## Write 500 columns, each having the payload bytes, to the row | |||
## Read the row back | |||
== The Results == | |||
=== Single Replication === | |||
It's quite apparent that the "append" method of database writing has a significant impact on the responsiveness of the server. While the number of columns in the payload has an affect on the write times, the size of the payload itself has minimal impact on performance. The read times, however small they are, are far more varied in value. Since the benchmarking is very simple in nature I can't pin down the exact cause of the erratic values. Since this test was performed with only single replication it's doubtful Cassandra's to blame, as only one Node need to be contacted and consulted. | |||
<center> | |||
<gallery caption="One Replication" widths="300px"> | |||
Image:ONE_REP-writes.jpg|Benchmark results for WRITING with one replication | |||
Image:ONE_REP-reads.jpg|Benchmark results for READING with one replication | |||
</gallery> | |||
</center> | |||
<br style="clear: both" /> | |||
=== Dobule Replication === | |||
When we add more replications into the mix we see a noted rise in both read and write times. What's more interesting to see is the statistical aberrations that are much more pronounced as you compound network latency and VPN overhead between the two nodes and ultimately the client. | |||
<center> | |||
<gallery caption="Two Replications" widths="300px"> | |||
Image:TWO_REP-writes.jpg|Benchmark results for WRITING with two replications | |||
Image:TWO_REP-reads.jpg|Benchmark results for READING with two replications | |||
</gallery> | |||
</center> | |||
<br style="clear: both" /> | |||
=== Triple Replication === | |||
With the third level of replication we can finally see the impact of the [[#Consistency|ALL]] consistency that my benchmark enforced. With these tests Cassandra had to ensure that every node had the latest update for the test row before the client call could be completed, which quite obviously has impacted both reading and writing for this series of tests. | |||
<center> | |||
<gallery caption="Three Replications" widths="300px"> | |||
Image:THREE_REP-writes.jpg|Benchmark results for WRITING with three replications | |||
Image:THREE_REP-reads.jpg|Benchmark results for READING with three replications | |||
</gallery> | |||
</center> | |||
<br style="clear: both" /> | |||
= Conclusions = | |||
Despite the statistical aberrations (which could have been caused by any number of factors) getting the components to talk together was mostly effortless. As I mentioned previously the implementation would most likely gain a significant performance boost by not relying on a third party for the VPN connection mediation. Not relying on a third party for connection permissions would also greatly increase the security and reliability on external resources. | |||
As a distributed database Cassandra certainly has potential, but I feel that the project is still too young to be completely reliable for the ideal goal of my project. It was only recently that the project introduced both indexes and paged results, something that I'd expect would be a higher (or more common sense) priority. | |||
To conclude, I do believe that this idea has merit and has a potential for further development but would require more planning and forethought before a proper implementation would be ready. | |||
=References= | |||
# [http://oreilly.com/catalog/0636920010852| Cassandra: The definitive guide] by Eben Hewitt © 2011 | |||
# [http://hamachi.cc| Hamachi homepage] |
Latest revision as of 19:47, 5 March 2011
In the beginning
The Internet has seen remarkable growth over the last few years, both technologically and socially. The demand for real-time information has increased at exponential rates and has put existing information systems to the test. For this paper I decided to look at two new software projects that have emerged over the last few years: Cassandra and Hamachi. Cassandra is a distributed database that evolved from the needs of websites such as Twitter and Facebook, whose need for frequent and short updates was too taxing on standard RDBMSs. Hamachi, which is in part a commercial project now, started as an open-source project aimed at creating zero-config VPNs. The project is now part of LogMeIn but is still free to use for non-commercial purposes.
In normal implementation circumstances you would not find these two projects paired together, but the concept of having a distributed database over a reasonably secure connection was one I thought was worth exploring. Over the course of this paper I'll discuss both projects in a reasonable amount of detail before detailing my implementation experiment and experience.
The Idea
My idea is to explore the feasibility of a near zero-config secure distributed database. Fully realized the idea of having a custom distribution of Linux that users could install on their hardware and be automatically joined onto a database distributed across thousands of machines and thousands of users.
Cassandra
Cassandra is a distributed, decentralized, scalable and fault tolerant database system (or at least it claims to be) currently under development by the Apache foundation. It shares some features from both the Bigtable and Dynamo projects, but has more focus on decentralization. It sports a tuneable consistency and replication system which, together with a flexible schema system, can quickly adapt to a site or project's growing needs. Well, enough salesman talk, let's get to the details.
Over the course of this subsection I'll be covering some of the basic concepts of Cassandra and how they contribute to the "bigger picture", as well as some implementation and installation details.
The Data Model
Cassandra takes a different approach compared to RDBMSs when it comes to how data is conceptualized and managed. In a standard RDBMS, data is normalized into a series of tables with a set number of columns. For the most part, the types and number of these columns will only rarely change as the need arises. For a later analogy, we'll refer to this model as a "Narrow Row" model. Cassandra operates on a system of Column Families which are analogous to a tables in an RDBMS, but is much more flexible. Column Families (hereafter referred to as CFs) are a loose grouping of common data keyed by some value for each row in question and are grouped together into Keyspaces. However, as opposed to the static feel of a RDBMS table, the number of columns or even the types of columns can and will change under Cassandra. That isn't to say there is no form of schema in Cassandra, because there is a simple type-enforcement schema system in place for each CF. To complete the analogy, the model for Cassandra can be seen as "Wide Row", with the number of columns growing however you please. Different rows in the same CF can even have a different number of columns. Internally, Cassandra uses a "always appending" model when writing data to disk, similar to Google's Bigtable or GFS.
The downside to such a data model is that Cassandra has no actual query language. There is no equivalent to SQL in Cassandra. There is also no support for referential integrity, either. With such circumstances maintaining data normalization while keeping processing time to a minimum is extremely difficult and is actively frowned upon. That's right, data duplication is encouraged when working with Cassandra. Luckily, the latest version (0.7.0 as of this writing) has introduced indexes to the meta-data that can be assigned to a column. This allows for basic boolean logic queries can be constructed to filter results better.
Scaling
While Cassandra can operate on a single machine the power lies in setting up a Cassandra cluster. Each instance is referred to as a node within the cluster. In Cassandra nomenclature the nodes form a ring and divide the keyspace amongst all of the nodes based on a specified partitioner. There are a number of partitioners available, including some that take physical location into account.
Fault Tolerance
Cassandra employs two protocols that make up it's fault tolerance system, Gossip and Handoffs. Gossip is an extension of the usual Heartbeat model of monitoring other servers, and involves a full TCP style SYN/ACK/SYN+ACK process to verify the alive-ness of another node in the ring. When a node attempts to contact another and fails, it "Gossips" this fact to the others. Using a special accrual method for detecting downed nodes the ring can keep track of problem areas and adjust accordingly. The second half of this fault tolerance suite is the Handoff protocol. When a write destined for a downed node is received by the ring, other nearby nodes will accumulate and keep these entries until the downed node is restored. During startup a node will check if any outstanding handoff messages are destined for it and import them promptly.
Replication
One of the nice features of Cassandra is a built-in Replication system. When a Keyspace is created the replication factor dictates how many copies of column data will be spread out over the ring. When writing or reading data to the nodes the clients can specify the desired consistency level that is to be observed relative to the Keyspace's replication factor. Like most distributed databases, Cassandra purports eventual consistency.
Consistency
- ONE consistency
- The client will block until the first node available responds to the request.
- QUORUM consistency
- The client will block until a majority of nodes agree on the data to return.
- ALL consistency
- The client will block until all nodes would return the same data
I'll give an example of the impacts of the various replication levels later in my implementation report.
Other options
Cassandra shares some common ground with a number of other projects:
- Bigtable
- Dynamo
- Riak
- CouchDB
Hamachi
Hamachi is a project aimed at easing the complication of creating and managing a Virtual Private Network. VPNs have historically been used to join external machines onto private networks. A travelling salesman or branch office might use one to gain access to a company's internal resources over possibly insecure lines. With a properly configured VPN encrypted traffic is tunneled over an insecure connection without concern that the contents could be inspected enroute. Hamachi has its origins in the modern playing of classic-era games that don't support WAN based gameplay. By creating a VPN with friends the game would be unable to tell that the machines were not all physically together.
Caveat
While the original implementation of Hamachi2 was open source the project is currently part of a commercial vendor called LogMeIn. The reason I chose to use this project was simply to fulfill a need for a quick VPN setup with a minimal amount of fuss. The performance and security of my implementation project would undoubtably be improved by an open source and mediator-free VPN solution such as OpenVPN. Because of the closed source nature of Hamachi I won't be able to comment much about the implementation details, it was simply a means to an end.
The Basics
Once the Hamachi client is installed, any client can simply create a network for others to join. Each client is assigned an address in the 5.0.0.0/8 IP range, which up until November 2010 was previously un-routable. This range was selected so as to not interfere with existing local network ranges. The client then, with the assistance of a central server, creates secure tunnels to every other client in the VPN. These tunnels support both TCP and UDP traffic, as well as broadcasting.
The Project
My idea was to combine these two separate projects together to create the semblance of a secure distributed database. In normal circumstances Cassandra nodes are expected to be located in a closed private network or to have a difficult-to-maintain VPN set up for them exclusively.
The Setup
For the purposes of this project I've created a demonstration project that tests the impact of both the inherent overhead from the VPN as well as the latency of physical distribution. The nodes are defined as follows:
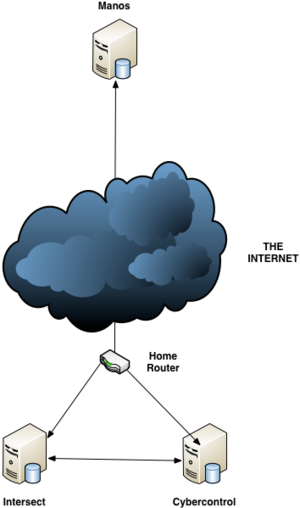
The Nodes
- Local Network
- Intersect (running OSX 10.6)
- Cybercontrol (running Ubuntu 10.10)
- Remote Network
- Manos (running Debian Squeeze)
All intra-node communication was over the VPN connection and the benchmarks were run through an API server running on Intersect. The Internal and External networks were separated physically by around 30km of prime Canadian internet.
Configuring Cassandra
I installed Cassandra 0.7 using the official Debian repository for both Cybercontrol and Manos. Both of these servers were configured to use Intersect as the seed node, the node (or nodes) that would be responsible for bootstrapping new nodes joining the ring. All the configuration that was required to get the nodes talking was to use the Hamachi IP assigned to Intersect and that was it.
The Schema
I used the following schema for the benchmarking. In it I define 3 keyspaces with 1 column family each. The primary difference between the three keyspaces is the replication factor assigned to each one. The keyspaces are:
- ONE_REP
- Single replication keyspace with a column family named
Somedata
- TWO_REP
- Dual replication keyspace with a column family named
MoreData
- THREE_REP
- Triple replication keyspace with a column family named
EvenMoreData
This schema is in YAML format and is compatible with Cassandra 0.7 only.
keyspaces: - column_families: - column_type: Standard comment: '' compare_with: org.apache.cassandra.db.marshal.BytesType default_validation_class: org.apache.cassandra.db.marshal.BytesType gc_grace_seconds: 864000 key_cache_save_period_in_seconds: 3600 keys_cached: 200000.0 max_compaction_threshold: 32 memtable_flush_after_mins: 60 min_compaction_threshold: 4 name: Somedata read_repair_chance: 1.0 row_cache_save_period_in_seconds: 0 rows_cached: 0.0 name: ONE_REP replica_placement_strategy: org.apache.cassandra.locator.SimpleStrategy replication_factor: 1 - column_families: - column_type: Standard comment: '' compare_with: org.apache.cassandra.db.marshal.BytesType default_validation_class: org.apache.cassandra.db.marshal.BytesType gc_grace_seconds: 864000 key_cache_save_period_in_seconds: 3600 keys_cached: 200000.0 max_compaction_threshold: 32 memtable_flush_after_mins: 60 min_compaction_threshold: 4 name: MoreData read_repair_chance: 1.0 row_cache_save_period_in_seconds: 0 rows_cached: 0.0 name: TWO_REP replica_placement_strategy: org.apache.cassandra.locator.SimpleStrategy replication_factor: 2 - column_families: - column_type: Standard comment: '' compare_with: org.apache.cassandra.db.marshal.BytesType default_validation_class: org.apache.cassandra.db.marshal.BytesType gc_grace_seconds: 864000 key_cache_save_period_in_seconds: 3600 keys_cached: 200000.0 max_compaction_threshold: 32 memtable_flush_after_mins: 60 min_compaction_threshold: 4 name: EvenMoreData read_repair_chance: 1.0 row_cache_save_period_in_seconds: 0 rows_cached: 0.0 name: THREE_REP replica_placement_strategy: org.apache.cassandra.locator.SimpleStrategy replication_factor: 3
The Benchmark
For the purposes of this experiment I wrote a simple script to test the responsiveness of a client reading and writing to each of the three keyspaces defined above. Each read or write performed by the script was done with the ALL consistency level, meaning that the call will block until all possible replications have the latest data.The benchmark script ran the following stages with various payload sizes 50 times, averaging the response time for each step:
- Stage 1
- Remove the test row if it already exists
- Write a single column with the payload bytes to the row
- Read the row back
- Stage 2
- Remove the test row if it already exists
- Write 50 columns, each having the payload bytes, to the row
- Read the row back
- Stage 3
- Remove the test row if it already exists
- Write 200 columns, each having the payload bytes, to the row
- Read the row back
- Stage 4
- Remove the test row if it already exists
- Write 500 columns, each having the payload bytes, to the row
- Read the row back
The Results
Single Replication
It's quite apparent that the "append" method of database writing has a significant impact on the responsiveness of the server. While the number of columns in the payload has an affect on the write times, the size of the payload itself has minimal impact on performance. The read times, however small they are, are far more varied in value. Since the benchmarking is very simple in nature I can't pin down the exact cause of the erratic values. Since this test was performed with only single replication it's doubtful Cassandra's to blame, as only one Node need to be contacted and consulted.
- One Replication
-
Benchmark results for WRITING with one replication
-
Benchmark results for READING with one replication
Dobule Replication
When we add more replications into the mix we see a noted rise in both read and write times. What's more interesting to see is the statistical aberrations that are much more pronounced as you compound network latency and VPN overhead between the two nodes and ultimately the client.
- Two Replications
-
Benchmark results for WRITING with two replications
-
Benchmark results for READING with two replications
Triple Replication
With the third level of replication we can finally see the impact of the ALL consistency that my benchmark enforced. With these tests Cassandra had to ensure that every node had the latest update for the test row before the client call could be completed, which quite obviously has impacted both reading and writing for this series of tests.
- Three Replications
-
Benchmark results for WRITING with three replications
-
Benchmark results for READING with three replications
Conclusions
Despite the statistical aberrations (which could have been caused by any number of factors) getting the components to talk together was mostly effortless. As I mentioned previously the implementation would most likely gain a significant performance boost by not relying on a third party for the VPN connection mediation. Not relying on a third party for connection permissions would also greatly increase the security and reliability on external resources.
As a distributed database Cassandra certainly has potential, but I feel that the project is still too young to be completely reliable for the ideal goal of my project. It was only recently that the project introduced both indexes and paged results, something that I'd expect would be a higher (or more common sense) priority.
To conclude, I do believe that this idea has merit and has a potential for further development but would require more planning and forethought before a proper implementation would be ready.
References
- Cassandra: The definitive guide by Eben Hewitt © 2011
- Hamachi homepage